Significance Level vs Confidence level vs Confidence Interval
- Stephanie Glen
- 16 jul 2021
- 5 Min. de lectura
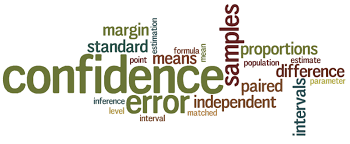
You may have figured out already that statistics isn't exactly a science. Lots of terms are open to interpretation, and sometimes there are many words that mean the same thing—like "mean" and "average"—or sound like they should mean the same thing, like significance level and confidence level.
Although they sound very similar, significance level and confidence level are in fact two completely different concepts. Confidence levels and confidence intervals also sound like they are related; They are usually used in conjunction with each other, which adds to the confusion.
However, they do have very different meanings.
In a nutshell, here are the definitions for all three.
Significance level: In a hypothesis test, the significance level, alpha, is the probability of making the wrong decision when the null hypothesis is true.
Confidence level: The probability that if a poll/test/survey were repeated over and over again, the results obtained would be the same. A confidence level = 1 - alpha.
Confidence interval: A range of results from a poll, experiment, or survey that would be expected to contain the population parameter of interest. For example, an average response. Confidence intervals are constructed using significance levels / confidence levels.
In the following sections, I'll delve into what each of these definitions means in (relatively) plain language.
Confidence level vs Confidence Interval
When a confidence interval (CI) and confidence level (CL) are put together, the result is a statistically sound spread of data. For example, a result might be reported as "50% ± 6%, with a 95% confidence". Let's break apart the statistic into individual parts:
The confidence interval: 50% ± 6% = 44% to 56%
The confidence level: 95%
Confidence intervals are intrinsically connected to confidence levels. Confidence levels are expressed as a percentage (for example, a 90% confidence level). Should you repeat an experiment or survey with a 90% confidence level, we would expect that 90% of the time your results will match results you should get from a population. Confidence intervals are a range of results where you would expect the true value to appear.
For example, you survey a group of children to see how many in-app purchases made a year. Your test is at the 99 percent confidence level and the result is a confidence interval of (250,300). That means you think they buy between 250 and 300 in-app items a year, and you're confident that should the survey be repeated, 99% of the time the results will be the same.
Let's delve a little more into both terms.
1. The Confidence Interval
This Gallup poll states both a CI and a CL. The result of the poll concerns answers to claims that the 2016 presidential election was "rigged", with two in three Americans (66%) saying prior to the election "...that they are "very" or "somewhat confident" that votes will be cast and counted accurately across the country." Further down in the article is more information about the statistic: “The margin of sampling error is ±6 percentage points at the 95% confidence level."
Let's take the stated percentage first. The "66%" result is only part of the picture. It's an estimate, and if you're just trying to get a general idea about people's views on election rigging, then 66% should be good enough for most purposes like a speech, a newspaper article, or passing along the information to your Uncle Albert, who loves a good political discussion. However, you might be interested in getting more information about how good that estimate actually is. For example, the real estimate might be somewhere between 46% and 86% (which would actually be a poor estimate), or the pollsters could have a very accurate figure: between, say, 64% and 68%. That spread of percentages (from 46% to 86% or 64% to 68%) is the confidence interval. But how good is this specific poll? The answer in this line:
“The margin of sampling error is ±6 percentage points…"
What this margin of error tells us is that the reported 66% could be 6% either way. So our confidence interval is actually 66%, plus or minus 6%, giving a possible range of 60% to 72%.
2. The Confidence Level
Again, the above information is probably good enough for most purposes. But, for the sake of science, let's say you wanted to get a little more rigorous. Just because on poll reports a certain result, doesn't mean that it's an accurate reflection of public opinion as a whole. In fact, many polls from different companies report different results for the same population, mostly because sampling (i.e. asking a fraction of the population instead of the whole) is never an exact science.
To make the poll results statistically sound, you want to know if the poll was repeated (over and over), would the poll results be the same? Enter the confidence level. The confidence level states how confident you are that your results (whether a poll, test, or experiment) can be repeated ad infinitum with the same result. In a perfect world, you would want your confidence level to be 100%. In other words, you want to be 100% certain that if a rival polling company, public entity, or Joe Smith off of the street were to perform the same poll, they would get the same results. But this is statistics, and nothing is ever 100%; Usually, confidence levels are set at 90-98%.
For this particular example, Gallup reported a " 95% confidence level,” which means that if the poll was to be repeated, Gallup would expect the same results 95% of the time.
A 0% confidence level means you have no faith at all that if you repeated the survey that you would get the same results. In fact, you're sure the results would be completely different.
A 100% confidence level means there is no doubt at all that if you repeated the survey you would get the same results. The results would be repeatable 100% of the time.
Confidence level vs Significance Level
Above, I defined a confidence level as answering the question: "...if the poll/test/experiment was repeated (over and over), would the results be the same?" In essence, confidence levels deal with repeatability. Significance levels on the other hand, have nothing at all to do with repeatability. They are set in the beginning of a specific type of experiment (a "hypothesis test"), and controlled by you, the researcher.
The significance level (also called the alpha level) is a term used to test a hypothesis. More specifically, it's the probability of making the wrong decision when the null hypothesis is true. In statistical speak, another way of saying this is that it's your probability of making a Type I error.
Constructing Confidence Intervals with Significance Levels
Using the normal distribution, you can create a confidence interval for any significance level with this formula:
sample statistic ± z*(standard error)
(z* = multiplier)
Confidence intervals are constructed around a point estimate (like the mean) using statistical table (e.g. the z-table or t-table), which give known ranges for normally distributed data. Normally distributed data is preferable because the data tends to behave in a known way, with a certain percentage of data falling a certain distance from the mean.
For example, a point estimate will fall within 1.96 standard deviations about 95% of the time.
Some opinions expressed in this article may be those of a guest author and not necessarily Analytikus.
From: https://www.datasciencecentral.com/profiles/blogs/significance-level-vs-confidence-level-vs-confidence-interval